News Nugget
Why this Matters
It’s difficult to be grateful for something that never happened. In the case of flooding, it’s hard to appreciate the decision-making and operations that went into preventing a flood, because the flood never happened. Human emotions work not unlike the FbF model, taking information from the past and constructing emotions based on predictions about the future. How can we use our growing knowledge of the roots of human emotion to fully realize the benefits of predictive computation and analytics?
Quick Takes
In honor of International Water Day, this morning is all about water. It makes up over 50% of our bodies and covers 71% of the earth’s surface. It has the softness to bathe a child, the power to carve from rock the Grand Canyon, and the lethal force to kill millions in the form of tsunamis and floods.
If you like this, please forward to three friends. If you received this message from a friend, sign up for the newsletter here.
Each year in West Africa floods take lives dozens of lives, displace thousands of families, and destroy tens of thousands of hectares of cropland. The plus side of all this water is that it has enormous potential for electricity production, making hydropower plants keys to economic security and growth in the region.
The dams, however, take the destructive power of floods out of God’s hands and into human responsibility. Dam operators have difficult jobs, balancing the need to maximizing electricity production with appropriately evaluating flood risk and releasing water early (losing electricity) in order to prevent flooding.
Human Problems: Crying Wolf, Accountability and Risk
The need to balance saving your job with saving lives is a difficult decision faced not only by dam operators; it is an industry-wide problem for those who provide humanitarian aid.
In the face of weather emergencies, government officials are hesitant to pull the trigger on fund disbursement or evacuation protocols for fear of “crying wolf”, receiving criticism and and budget cuts for false alarms. As a result, they often wait until an environmental disaster is obvious and imminent before issuing evacuation protocols, at which point it’s usually too late.
Forecast-based financing (FbF), which uses computational algorithms to predict weather emergencies, assigns risk probabilities and recommends action such as fund disbursement or evacuation, provides documented risk analysis outside the realm of human judgment and blame. Every step in the decision-making is recorded and accountable, allowing iterative adjustments of the algorithms that evaluate risk and determine action.
Computer Problems: Designing for Human Factors in the Data Landscape
Machine learning was considered for this project, but machines can’t learn without data. In designing an effective computational solution for a problem, you have to deeply understand the human factors that shape the data landscape.
For example, the technology infrastructure in Togo is not yet developed enough for automated hydrometeorological data collection, which means flood prediction data needs to be manually collected.
Herman Dolder therefore designed the ‘functional estimation’ tool or FUNES to heavily rely on a time series of one simple measurement: rainfall. The model acts as a ‘village elder’, by remembering past information and finding the most similar situation and applying some extrapolations in order to make a prediction for future flood risk. This gives dam operators data-backed reasoning to handle flood events as opposed to following a set of predefined standard operating procedures.
Human-Computer Solution: A Computer Serves as Togolese ‘Village Elder’
In 2015 the FUNES model was tested when the Togolese government trained 1,000 Red Cross volunteers to collect water level data downstream the Nangbeto Dam on the Mono River and enter it into the FUNES tool via text message.
The results shown below show that the algorithm did in fact reduce peak flooding from a single large incident of 900 cubic meters of outflow to two smaller incidents of 800 cubic meters. This reduction in peak flow is enough to prevent water from escaping its channels and damaging crop land and homes, while maximizing for electricity production at the dam.
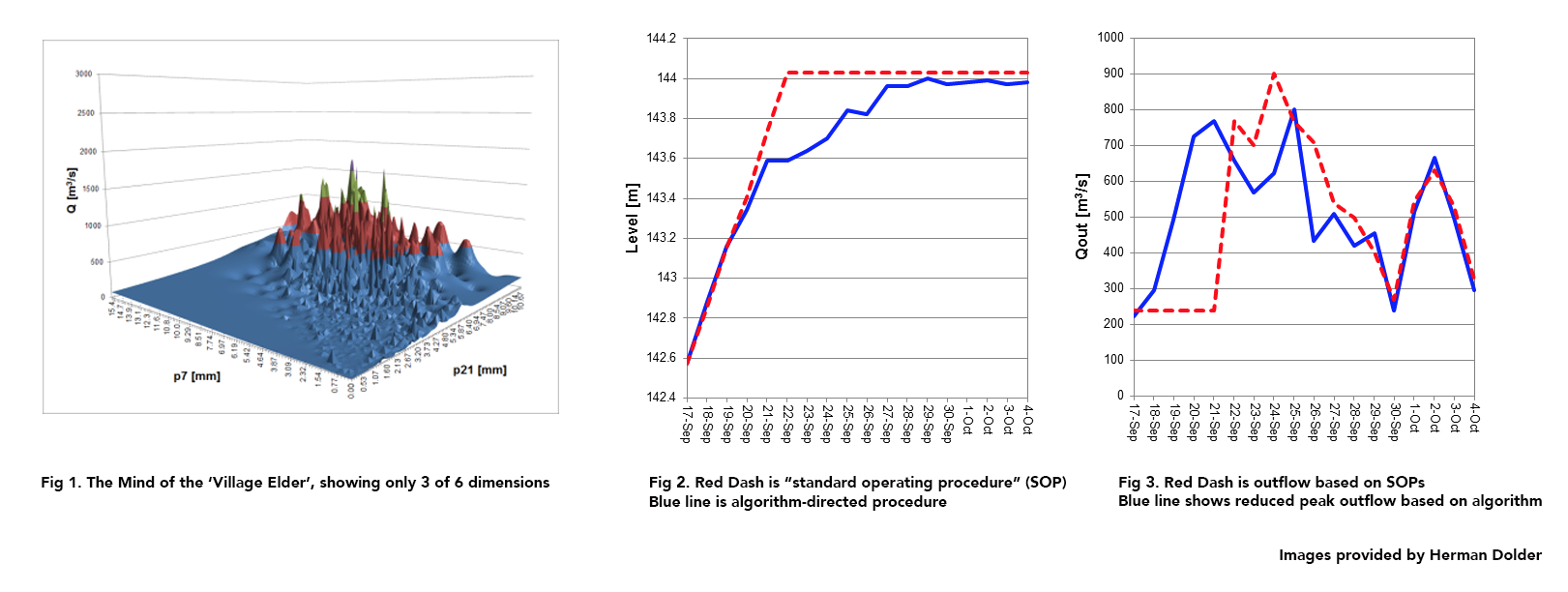
The model however, is only as good as the data provided. Dolder points out that “on a nice day you get 20 inputs [from volunteers], when it rains you have zero”, and sometimes people guess the rainfall instead of going to the river to measure.
“On a nice day you get 20 inputs [from volunteers], when it rains you have zero”, and sometimes people guess the rainfall instead of going to the river to measure.
However, with new satellites going into orbit dedicated to geo-monitoring that have unprecedented visual precision in combination with low-cost weather stations built on Raspberry Pi for under $100 (as opposed to current weather stations billed at several thousand), the problem of human error and too little data are likely to be reduced — opening the door for applied machine learning in climate science and forecast-based financing.
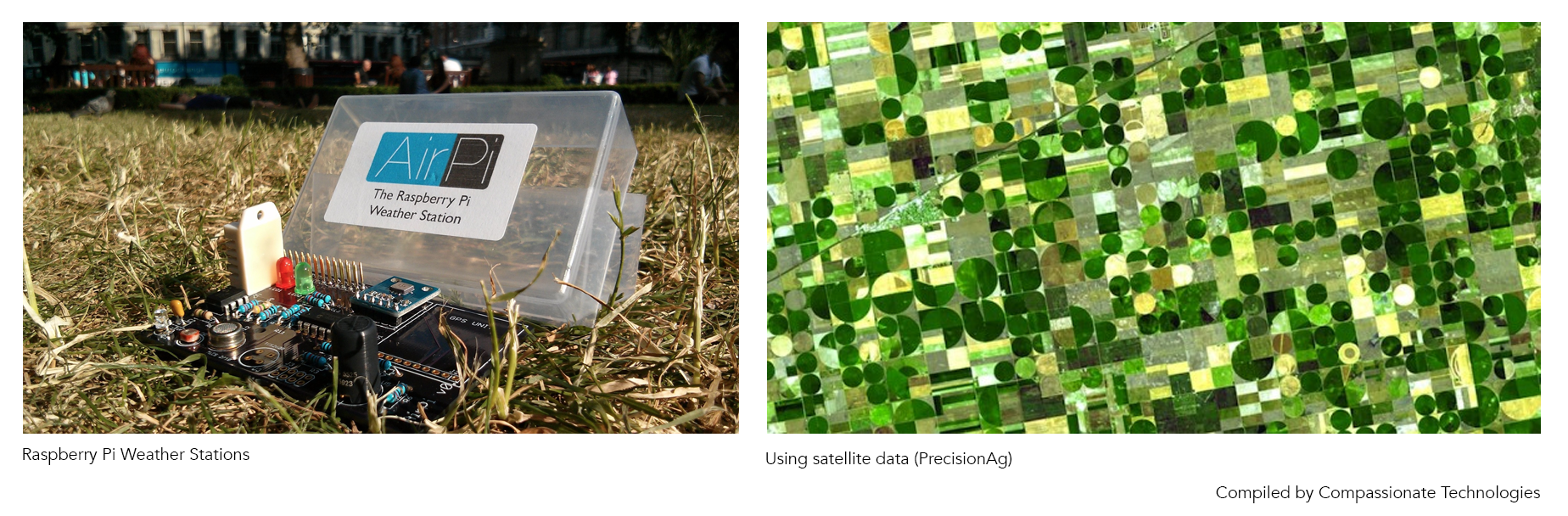
News Nugget
Why this Matters
It’s difficult to be grateful for something that never happened. In the case of flooding, it’s hard to appreciate the decision-making and operations that went into preventing a flood, because the flood never happened. Human emotions work not unlike the FbF model, taking information from the past and constructing emotions based on predictions about the future. How can we use our growing knowledge of the roots of human emotion to fully realize the benefits of predictive computation and analytics?